We’re seeing AI chatbots popping up across industries trending fast, reducing workloads, and helping teams move quicker. AI chatbots are everywhere, and it’s got business leaders thinking.
“Should we also implement one?”
But before you even go there, ask this. “What’s the AI Chatbot ROI?”
ROI for AI in customer service is tricky to define. As, implementing AI chatbots for customer service is not just about cutting costs. It’s about fewer support tickets, faster resolutions, improved CSAT, and even some indirect revenue gains.
Grand View Research reports that the AI chatbot market is projected to hit $9.56 billion by 2025, and climb to $27.29 billion by 2030, growing at a CAGR of 23.3%. This kind of growth indicates that AI chatbots are becoming more like a core part for businesses aiming to boost efficiency and deliver better service, rather than just being a bonus feature.
And it’s not just limited to tech companies. Below is a chart showing how AI chatbots are being used across different industries.
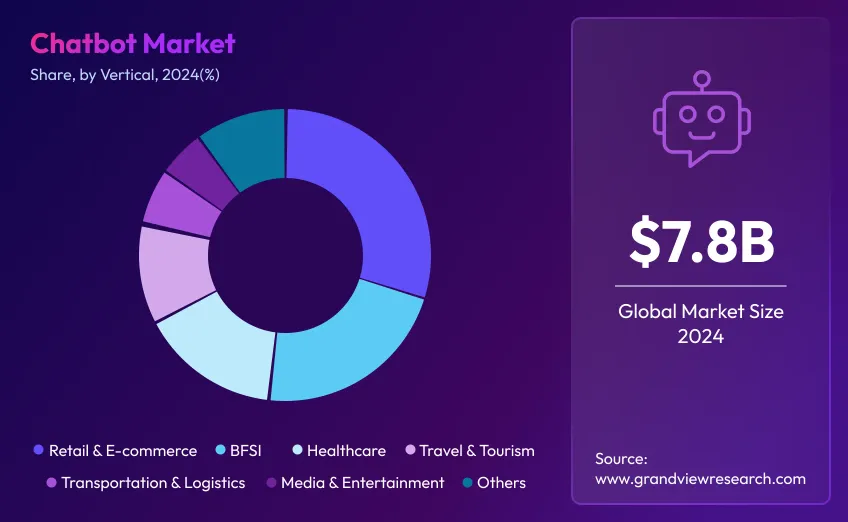
From banking to healthcare to retail, the usage of AI chatbots is spreading fast across industries.
Growth and time-saving sound good, but as you know, businesses need a wider lens.
Let's explore whether AI chatbot development services are really worth the investment or not?
Before that, let's get a quick brief of what is ROI in AI chatbots.
Overview: ROI in AI chatbots
ROI or Return on Investment (ROI) measures the value gained relative to the cost spent. When it comes to AI chatbots, ROI isn’t just about cutting expenses. It focuses more on improvements in efficiency, availability, customer satisfaction, and actionable insights.
For businesses adopting AI development services for chatbot, the return isn't only financial. It is about improving service quality and streamlining operations.
Rather than just a financial report, smart businesses use chatbot performance metrics to assess the ROI of AI chatbots and evaluate how the chatbot contributes to operational success.
ROI in AI chatbots vs traditional software
Aspect | AI Chatbot | Traditional software |
---|---|---|
Customer experience | Instant, 24/7 support, highly personalized AI | Limited availability, often lacks personalization |
Cost efficiency | Reduces operational costs, AI chatbot ticket deflection | Higher staffing costs, longer resolution times |
Operational efficiency | Automates tasks, integrates with chatbot CRM integration | Manual processes, less efficient |
Impact over time | Improves through machine learning, evolving performance | Static, doesn’t evolve with time |
To get an idea of return on investment first, let’s see what is invested in AI chatbot deployment.
Cost of chatbot deployment: Complete breakdown
A detailed look at the costs involved in deploying an AI chatbot can help businesses better understand the investment and evaluate its true value.
One-time vs ongoing expenses
Expense type | One-time costs | Ongoing costs |
---|---|---|
Development | Initial setup and development of the AI chatbot | Regular updates and improvements |
Training data | Data collection and preprocessing | Ongoing data updates and training |
Integrations | API connections and integration setup | Maintenance of integrations |
Build vs buy cost trade-offs
Option | Cost consideration | Pros | Cons |
---|---|---|---|
Pre-built tools | Lower upfront costs | Faster deployment, less complex | Limited customization |
Custom bots designed for business logic | Higher upfront costs | Tailored to business needs | Longer deployment time, higher cost |
Hidden or indirect costs to consider
- Internal training required for staff to operate and manage the chatbot
- Downtime during rollout or system transition periods
- Opportunity costs if the chatbot doesn't meet real user needs, leading to inefficiencies
- Data security and compliance costs
Key metrics to track chatbot performance and ROI
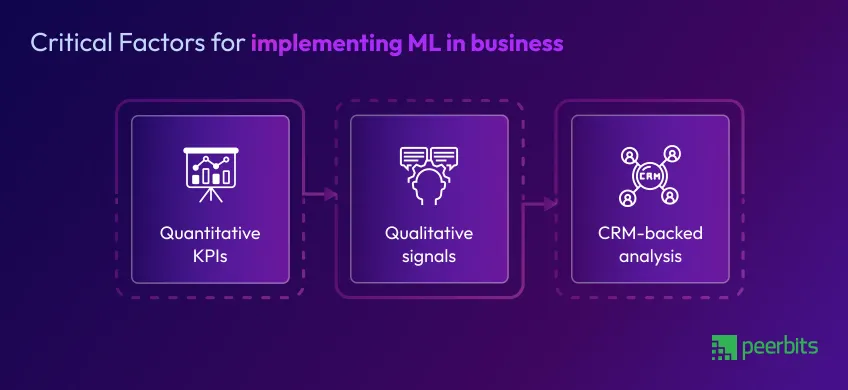
To measure the effectiveness of AI chatbots in customer service, it’s essential to track both quantitative and qualitative performance metrics. These metrics help gauge not just ROI but also the chatbot’s influence on customer experience and operational efficiency.
Quantitative KPIs:
- Average response time: Measures how quickly the chatbot responds to user queries.
- Resolution rate: Tracks the percentage of issues fully resolved by the chatbot.
- Ticket deflection rate: Assesses how many queries are handled by the chatbot, reducing the need for human agents.
- Cost per resolved query: Calculates the average cost to resolve each customer issue using the chatbot.
- Bot containment rate: Measures the percentage of issues resolved by the chatbot without human intervention.
Qualitative signals:
- CSAT and NPS from bot-handled interactions: Tracks customer satisfaction and net promoter score for chatbot-driven conversations.
- Session duration and dropout points: Analyzes how long customers engage with the chatbot and where they drop off in the conversation.
CRM-backed analysis:
- Before vs after data on ticket volume or revenue per user: Compares ticket volume or user revenue before and after the chatbot is implemented.
- CRM + chatbot integration helps show longitudinal ROI: Tracks long-term ROI using data from CRM combined with chatbot performance over time.
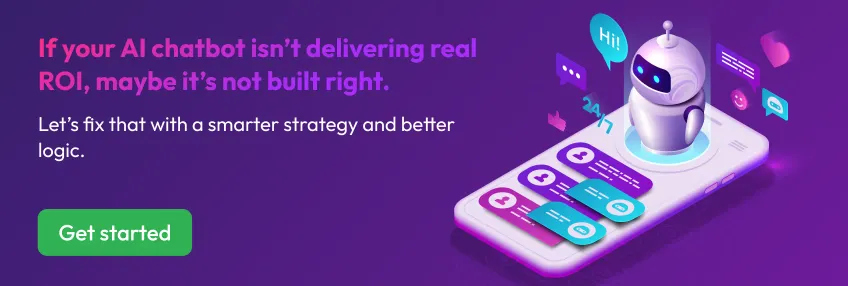
ROI calculation frameworks: Turning data into decision-making
ROI in AI chatbot deployments is different from the standard formula. It needs to account for unique factors like saved hours, reduced support costs, and revenue growth.
The basic ROI formula adapted for AI chatbots:
- ROI = (Gain – Cost) / Cost
Where:
- Gain = Hours saved, support cost reduction, and revenue influenced by the chatbot.
- Cost = Total lifecycle cost (setup + maintenance + training).
Example:
Let’s say a business deploys an AI chatbot with an initial setup cost of $10,000. The ongoing maintenance cost is $2,000 per year, and after 1 year, the chatbot saves 500 hours of agent time (valued at $30 per hour) and reduces support costs by $5,000.
- Gain = (500 hours x $30) + $5,000 = $20,000 + $5,000 = $25,000
- Cost = Setup cost ($10,000) + Maintenance cost ($2,000) = $12,000
Using the formula:
ROI = ($25,000 – $12,000) / $12,000 = $13,000 / $12,000 = 1.08
This results in an ROI of 108% for the first year.
Custom ROI dashboards: Tools businesses use
- Salesforce, Zendesk, HubSpot, and custom dashboards can be used to track and visualize ROI data.
- Pairing metrics with timelines helps businesses evaluate chatbot performance trends and make timely adjustments with the help of essential AI tools for better & faster customer support.
How to know when it’s time to iterate?
If certain KPIs stall, user feedback declines, or engagement drops, it may be time to retrain or reposition the chatbot to meet evolving business goals.
What’s considered a “healthy” ROI in chatbot deployments?
There’s no one-size-fits-all benchmark for AI chatbot ROI. What’s considered “healthy” varies by use case, business goal, and how the chatbot is deployed with the help of AI development services.
Why context matters:
- A logistics company using chatbots for shipment tracking will calculate ROI differently than an e-commerce brand automating order FAQs.
- Fintech chatbots often involve higher compliance and complexity, shifting the ROI expectations.
It’s not just about numbers:
- A “healthy” ROI in chatbot deployments is defined by business goals.
- For some, it’s cost savings or response speed. For others, it’s about customer satisfaction, data insights, or lead qualification.
- The deeper the chatbot integration into existing systems (like CRM or support tools), the more impactful the return — even if it takes longer to reflect on paper.
Conclusion
Measuring AI chatbot ROI isn’t about ticking boxes. It’s about knowing whether your investment is actually helping saving time, reducing support load, or improving customer satisfaction.
From cost breakdowns to performance metrics and decision frameworks, every part of this process points toward one core idea. Are your AI chatbot deployments delivering real value?
You don’t need a perfect number to move forward. But you do need a clear view. ROI gives you that lens. And once you have it, chatbot implementation stops being a guess. It becomes a confident business decision.
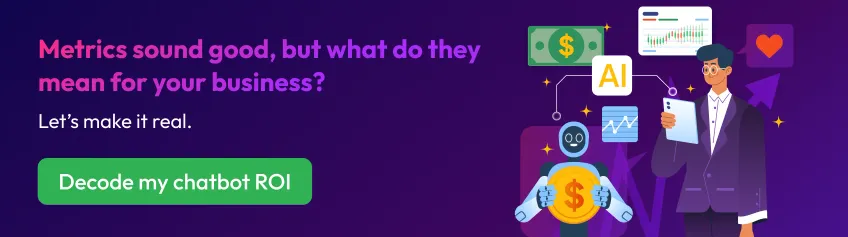