Many businesses today use analytics tools, yet issues like rising support volumes, falling satisfaction scores, and customer drop-offs continue to persist. The core problem usually isn’t data availability. It’s the way that information is processed, interpreted, and applied in high-pressure, real-time environments such as customer service.
Most businesses rely on traditional analytics to track and report performance. These systems are well-suited for structured, historical data. They offer useful summaries, periodic reports, and trend analysis. But when it comes to understanding fast-changing customer behavior or predicting what users need next, the limitations begin to show.
The need for real-time, context-aware decisions is leading more teams to explore smarter options. This is where the conversation around AI analytics vs traditional analytics becomes practical, focusing on which system better supports today’s customer service goals.
According to Statifacts, the global AI analytics market was valued at $29.15 billion in 2024 and is projected to cross $225 billion by 2034, growing at a CAGR of 22.7 percent. The numbers reflect what businesses are already seeing on the ground: modern problems require more adaptive analytics, not just more dashboards.
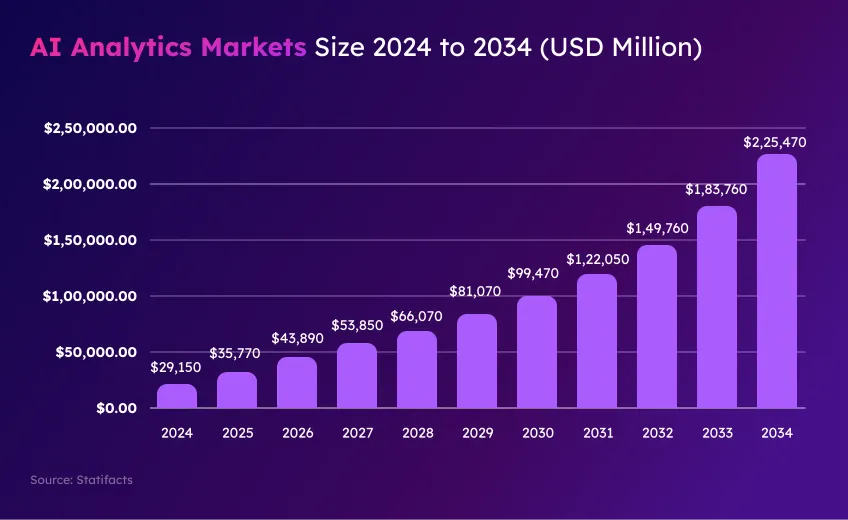
Before exploring any AI analytics solution, it’s important to understand what traditional analytics still does well and where it starts falling short.
Role of traditional analytics in customer operations
Traditional analytics forms the backbone of many customer service operations. It excels at processing structured, historical data but struggles with fast-changing, complex customer interactions..
What is traditional analytics built to do?
Traditional analytics works best with structured, historical data. It’s built to:
- Track core metrics like CSAT, FRT, and NPS.
- Analyze trends and support volume patterns.
- Apply fixed rules and filters to sort data.
- Generate reports at set intervals.
- Rely on manual effort to extract deeper insights.
Benefits of traditional analytics
Even now, traditional analytics has its place. It remains useful for:
- Periodic performance reviews and reporting.
- Simple implementation and maintenance.
- Linear workflows with predictable patterns.
Limitations of traditional analytics
Its limitations become clear in dynamic environments. It often:
- Can’t process real-time data or live customer inputs.
- Misses emotional signals or urgent context in conversations.
- Struggles with unstructured formats like voice or chat.
- Depends heavily on manual interpretation and follow-up.
Importance of AI analytics
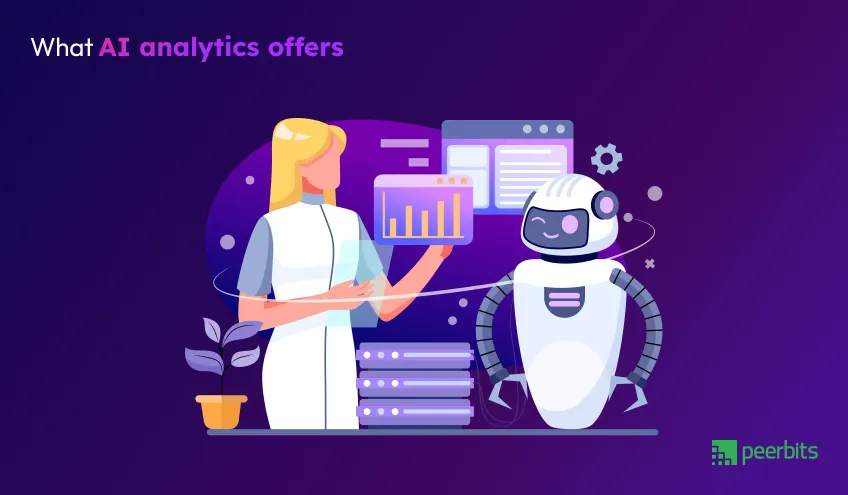
Traditional analytics focuses on what happened. AI analytics focuses on what’s happening and what’s likely to happen next. It doesn’t just process data; it interprets intent, emotion, and urgency across structured and unstructured inputs.
How it reads data differently
An AI analytics solution works beyond rigid datasets. It can:
- Process both structured and unstructured data sources.
- Learn from user behavior, not just tracked metrics.
- Detect patterns, sentiment shifts, and escalation risks.
- Forecast likely outcomes based on historical and real-time signals.
Practical AI features in customer service
AI analytics is already powering smarter service delivery through:
- Auto-prioritization of incoming tickets based on urgency.
- Sentiment-based routing to match tone with the right agents.
- Early detection of customer dissatisfaction or exit risks.
- Conversation summarization for faster agent wrap-ups.
- Performance feedback based on live and historical chats.
Real-time action, not just analysis
Unlike traditional models, AI systems act as the moment unfolds:
- Recommend next-best actions while chats are ongoing.
- Escalate emotionally charged cases automatically.
- Flag recurring issues across multiple conversations.
Traditional vs AI analytics: Detailed comparison
When evaluating AI analytics vs traditional analytics, it helps to break down how they function across common business needs. This table highlights where each model fits and why many teams are shifting toward AI-powered decision support.
Factor | Traditional analytics | AI analytics |
---|---|---|
Data type | Processes only structured data from forms, spreadsheets, or databases | Combines structured data with unstructured sources like chat logs, voice transcripts, emails |
Speed | Operates in batches with delayed reporting and lag in insight delivery | Works in real-time, offering live data interpretation and on-the-fly decision support |
Insights | Relies on human teams to extract and interpret patterns | Automatically detects context, trends, sentiment, and next steps without manual effort |
Use case fit | Best suited for generating reports and tracking KPIs over time | Fits dynamic environments where fast, informed action is needed—such as live customer support |
Personalization | Offers general trend analysis with no customer-level depth | Adapts to individual behaviors and provides predictive, context-aware recommendations |
Setup | Based on fixed rules and filters that require manual tuning | Uses self-learning models that adapt over time with less ongoing configuration |
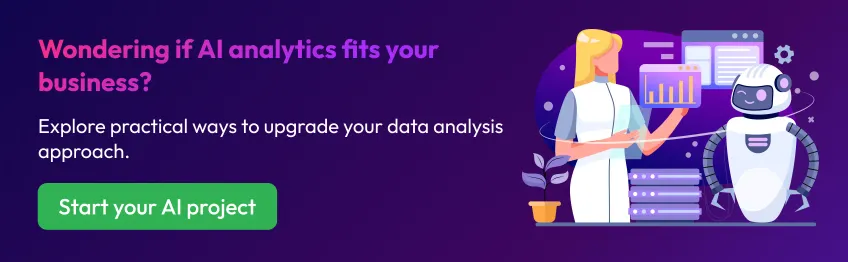
What this shift means for your support team?
The move from traditional data analysis to AI-based data analytics does more than change how reports look, it changes how support teams operate on a day-to-day level. With AI in customer service, teams gain faster visibility, fewer manual processes, and insights that actually support decision-making in real time.
Operational impact
- Tagging and triaging become automatic, so agents no longer lose time sorting requests manually.
- Complex cases get addressed faster, as AI surfaces urgency, context, and history instantly.
- Support and product teams stay better aligned, with shared visibility into recurring issues and customer feedback trends.
Team experience
- Agents feel more in control, as AI assists with suggestions and context without replacing their judgment.
- Managers gain a live pulse on performance, from sentiment trends to real-time queue monitoring.
- Performance reviews become more accurate, going beyond ticket counts to include behavioral patterns and resolution quality.
This change affects both the technology and the way teams work. An AI analytics solution helps support teams respond faster, focus on high-impact issues, and stay aligned with business goals.
Factors to consider before adopting AI analytics
Before moving toward any AI-powered analytics solution, it’s important to assess internal readiness, not just in terms of tools, but also in how data flows and decisions are made. A successful shift starts with asking the right questions.
Data readiness
- Customer data needs to be clean, connected, and accessible across systems like CRMs, helpdesks, and ticketing tools.
- Chat logs, behavior patterns, and case history must be available for AI models to learn and deliver meaningful insights.
Tool vs system thinking
- Adopting an AI tool alone doesn’t lead to smarter support unless it fits your business logic and workflows.
- AI analytics works best as part of a larger system, where data, people, and processes are aligned.
Soft costs to factor
- Training models takes time, especially when tuning them to your service patterns and customer behavior.
- Team habits may need to shift, with more trust in automation and AI-assisted decision-making.
- Workflow updates will be required, from how tickets are routed to how insights are used during performance reviews.
Tools & technologies for AI-powered and traditional analytics
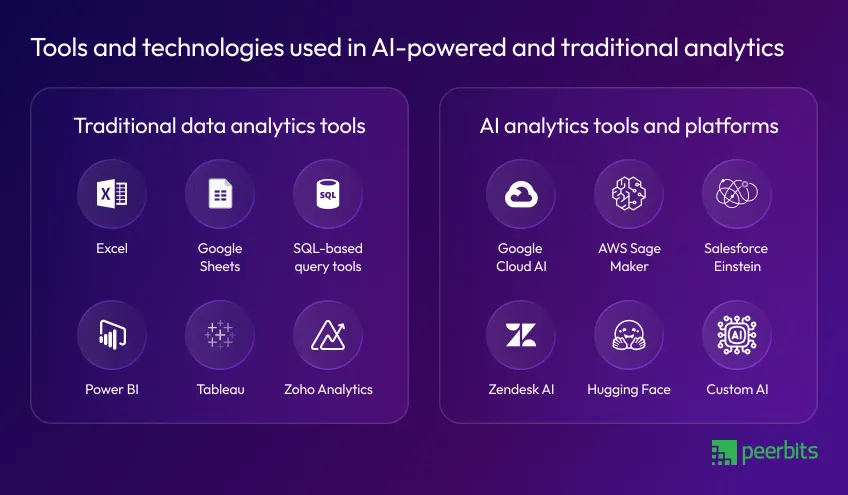
The effectiveness of any customer service strategy depends heavily on the right analytics stack. Whether you're working with traditional data analysis methods or exploring an AI analytics solution, the tools you choose shape how your business interprets, responds to, and benefits from data.
Traditional data analytics tools
Traditional data analytics relies on structured datasets and static logic. It's ideal for consistent reporting, but less effective in handling modern, multi-channel customer interactions.
- Excel, Google Sheets: Still used across departments for basic calculations and static reports.
- SQL-based query tools: Core to many traditional data analysis methods, enabling custom report generation from relational databases.
- Power BI, Tableau: Popular platforms for building dashboards using structured customer data.
- Zoho Analytics, Freshdesk Analytics: Examples of traditional data analytics tools used in customer service for tracking resolution times, ticket volume, and satisfaction scores.
These tools work well for business environments with stable workflows and predictable data trends. But their insights are limited to what has already happened, often requiring human effort to draw conclusions.
AI analytics tools and platforms
AI-based data analytics expands beyond structured inputs. These tools use machine learning techniques to process unstructured data, predict behaviors, and deliver live insights, key capabilities in modern customer service operations.
- Google Cloud AI, AWS SageMaker, Azure AI Studio: Platforms to build and manage large-scale AI models for real-time data processing.
- Salesforce Einstein, Zendesk AI, Intercom Fin: Platforms that integrate AI in customer service by offering sentiment detection, smart routing, and automated replies.
- Hugging Face, TensorFlow, PyTorch: Popular frameworks for building custom AI analytics tools that can support personalized insights, churn risk detection, and conversation summarization.
- Custom AI dashboards: Often built using NLP and ML to serve real-time business analytics and AI-driven decision support.
These AI-powered tools aren’t just reactive, they support proactive decisions. Whether it’s helping an agent during a live chat or flagging a negative trend early, AI advanced analytics helps teams stay one step ahead.
Real-world examples of AI-driven customer analytics
Many businesses are turning to AI analytics solutions to gain deeper customer insights, improve service quality, and make faster decisions. These AI-based data analytics tools help overcome limitations of traditional data analysis methods by processing complex, real-time information and unstructured data.
Amazon
- Challenge: Handling vast amounts of customer data to deliver personalized recommendations that drive sales and improve customer experience is complex with traditional data analytics tools.
- Solution: Amazon uses AI-powered analytics to analyze both structured and unstructured data in real time, enabling highly tailored product suggestions that adapt to changing customer preferences.
Netflix
- Challenge: Reducing customer churn and improving engagement by accurately predicting viewer preferences is difficult with static reporting methods.
- Solution: Netflix applies AI in customer service analytics to track behavior patterns and viewing habits, offering personalized content that keeps users engaged longer.
Spotify
- Challenge: Increasing user satisfaction requires understanding individual listening habits and moods beyond basic metrics.
- Solution: Spotify leverages AI-based data analytics to process complex user data and deliver personalized playlists that resonate with users’ tastes and emotions.
Comcast
- Challenge: Managing and prioritizing a high volume of customer support tickets while maintaining service quality can overwhelm traditional analytics systems.
- Solution: Comcast employs AI analytics tools to detect customer sentiment and prioritize tickets automatically, improving response times and support efficiency.
Key team roles for a high-impact AI analytics team
Building AI-based data analytics into customer operations goes beyond hiring data experts. It requires a team that understands both machine intelligence and real business workflows. AI integration of analytics should support day-to-day decisions without disrupting how your team works.
To develop AI tools for data analysis that are actually useful in fast-moving environments, you need:
- Product-focused professionals who translate insights into customer-impacting actions.
- UX observers who understand how real conversations unfold across channels.
- Infrastructure engineers who can support scalable, real-time systems that don’t break under pressure.
These roles ensure AI is supporting the business, not creating extra complexity.
What good partners bring?
Working with experienced AI consulting services or technical teams brings added value when they can:
- Connect fragmented data like CRM logs, tickets, and user interactions into usable formats.
- Train and refine models with ongoing feedback from live data.
- Build reliable systems that help AI-driven data analytics run in the background without constant attention.
- A strong system doesn’t require teams to work harder. It quietly supports smarter decisions through better analysis.
Conclusion
AI analytics vs traditional analytics highlights how businesses can gain deeper customer insights and faster decision-making by moving beyond traditional data analysis tools. While traditional data analytics still supports basic reporting and stable operations, it lacks real-time processing and the ability to handle unstructured data like chats or voice.
AI-based data analytics uses advanced analytics and AI tools for data analysis to deliver predictive, personalized outcomes. This makes AI in customer service more effective by enabling sentiment analysis, ticket prioritization, and early churn detection.
Before adopting AI analytics, businesses should assess data readiness and consider AI consulting services to ensure smooth integration with existing workflows. Ultimately, AI-powered analytics offers practical solutions that align with today’s dynamic customer experience needs, helping teams work smarter and deliver better results.
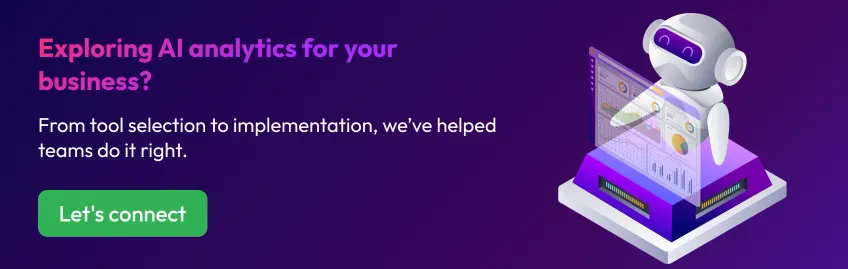
FAQ's
Adopting AI analytics doesn’t require starting from scratch. Many tools are modular and integrate easily. Costs depend on data complexity, automation scale, and build vs. partner choices.
Timelines vary, but most teams start seeing measurable improvements in operational insights or decision-making within 2–3 months of implementation, especially when using pre-trained models or industry-specific solutions.
Yes, most AI analytics platforms today offer intuitive dashboards and visualizations. With the right training and interfaces, product managers, marketers, and support leads can extract meaningful insights without deep technical knowledge.
AI systems often need access to large datasets, some of which may contain sensitive customer information. It’s important to choose platforms with strong encryption, role-based access control, and compliance with standards like GDPR or SOC 2.
If your current analytics feel reactive, your data is scattered across platforms, or you struggle to interpret customer behavior at scale, these are good signs that it’s time to consider AI-driven solutions.
These tools help interpret conversations, detect sentiment, predict churn, and even suggest next-best actions to agents during live interactions, resulting in faster, more relevant support.